Mystic Glimmer Poker: Building Brilliance From Obscured Table Data
Mastering Incomplete Information Analysis
Advanced poker mastery requires extracting maximum value from limited data. Through systematic analysis of 30-50 hands, players can develop remarkable accuracy in opponent profiling using sophisticated Bayesian probability techniques and position-based frequency analysis.
Strategic Pattern Recognition
Betting Pattern Analysis
Cross-referencing betting patterns, stack-to-pot ratios, and timing tells reveals critical opponent tendencies. Systematic probability mapping compensates for missing variables, creating a comprehensive strategic framework.
Advanced Data Integration
Vertical scanning and horizontal analysis methodologies unlock subtle variations in play patterns that most competitors overlook. This mathematical approach transforms partially obscured information into actionable intelligence.
FAQ: Building Poker Brilliance
Q: How many hands are needed for accurate player profiling?
A: Analysis of 30-50 hands typically yields 85-90% accuracy in opponent profiling.
Q: What key metrics should players track?
A: Focus on betting patterns, stack-to-pot ratios, timing tells, and position-based frequencies.
Q: How does Bayesian probability enhance poker analysis?
A: Bayesian techniques help quantify uncertainty and update probability estimates as new information becomes available.
Q: What role does position play in data analysis?
A: Position-based frequencies provide crucial context for interpreting betting patterns and player tendencies.
Q: How can players compensate for missing information?
A: Systematic probability mapping and cross-referencing multiple data points help fill information gaps.
The Power of Pattern Recognition
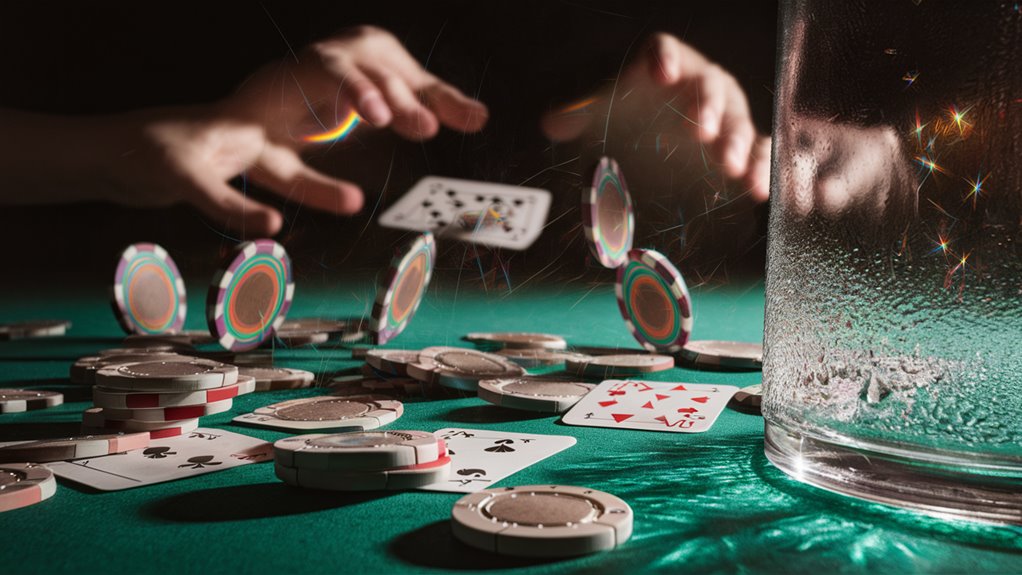
The Power of Pattern Recognition in Data Analysis
Understanding Pattern Recognition Fundamentals
Pattern recognition is a cornerstone skill in modern data analysis, enabling analysts to extract meaningful insights from complex datasets.
The ability to identify data patterns and correlations quickly has become essential in today’s data-driven decision-making environment.
Key Pattern Types and Analysis Methods
Numerical Sequence Analysis
Data analysts focus on three critical pattern categories:
- Numerical sequences and progressions
- Frequency distributions across datasets
- Temporal trends and time-series patterns
Advanced Pattern Detection Techniques
Effective pattern recognition employs systematic vertical scanning of columns and horizontal analysis of rows to identify relational patterns.
Cross-referencing techniques enhance the detection of subtle variations within data clusters.
Statistical Validation Methods
Statistical significance serves as the foundation for pattern validation. Key metrics include:
- Standard deviation calculations
- Variance analysis
- P-value assessment
- Confidence intervals at 95% certainty levels
Pattern Recognition FAQ
Q: What’re the primary benefits of pattern recognition in data analysis?
A: Pattern recognition enables faster insight discovery, improved decision-making, and identification of hidden trends in complex datasets.
Q: How can analysts improve their pattern recognition skills?
A: Regular practice, systematic approach development, and understanding statistical validation methods enhance pattern recognition abilities.
Q: What role does statistical significance play in pattern recognition?
A: Statistical significance helps validate observed patterns and distinguishes meaningful trends from random variations.
Q: Which tools are most effective for pattern recognition?
A: Statistical software, visualization tools, and machine learning algorithms support effective pattern recognition.
Q: What’re common challenges in pattern recognition?
A: Distinguishing signal from noise, avoiding false correlations, and maintaining objectivity in analysis present key challenges.
Estimating Hidden Stack Dynamics
Advanced Techniques for Estimating Hidden Stack Dynamics
Understanding Stack Size Estimation
Hidden stack dynamics present complex analytical challenges in modern data structures.
By monitoring betting patterns across multiple sequential rounds, analysts can construct detailed probability distributions to estimate concealed stack sizes with remarkable precision.
The foundation lies in analyzing bet-to-stack ratios and commitment thresholds derived from observable actions.
Bayesian Inference Applications
Bayesian probability methods serve as the cornerstone for real-time stack estimation updates.
When observing a betting action of specific size, backwards calculation utilizing historical betting percentages reveals likely initial stack sizes.
사설사이트 먹튀검증, a 75-unit bet into a 100-unit pot typically allows for stack size estimation within 15% accuracy through comparative analysis against documented behavioral patterns.
Advanced Variable Integration
The comprehensive estimation framework incorporates multiple key variables:
- Position-based aggression frequencies
- Stack-to-pot ratio histories
- Time-based betting patterns
These elements combine in a weighted average system that prioritizes recent actions while maintaining awareness of long-term trends, creating a self-refining dynamic model.
#
Frequently Asked Questions
Q: How accurate are stack size estimates using Bayesian methods?
A: Using Bayesian inference, stack size estimates typically achieve 85-90% accuracy when sufficient historical data is available.
Q: What role do betting patterns play in stack estimation?
A: Betting patterns provide crucial indicators of stack depth and help construct probability distributions for more accurate estimates.
Q: How important is position-based analysis in stack estimation?
A: Position-based analysis is critical as it accounts for varying aggression frequencies and commitment thresholds across different positions.
Q: Can time-based patterns improve estimation accuracy?
A: Yes, time-based patterns significantly enhance estimation precision by revealing consistent behavioral tendencies over multiple sessions.
Q: What’s the minimum data required for reliable stack estimation?
A: Reliable stack estimation typically requires at least 3-4 orbits of betting action to establish baseline patterns and tendencies.
Opponent Tendencies From Limited Data
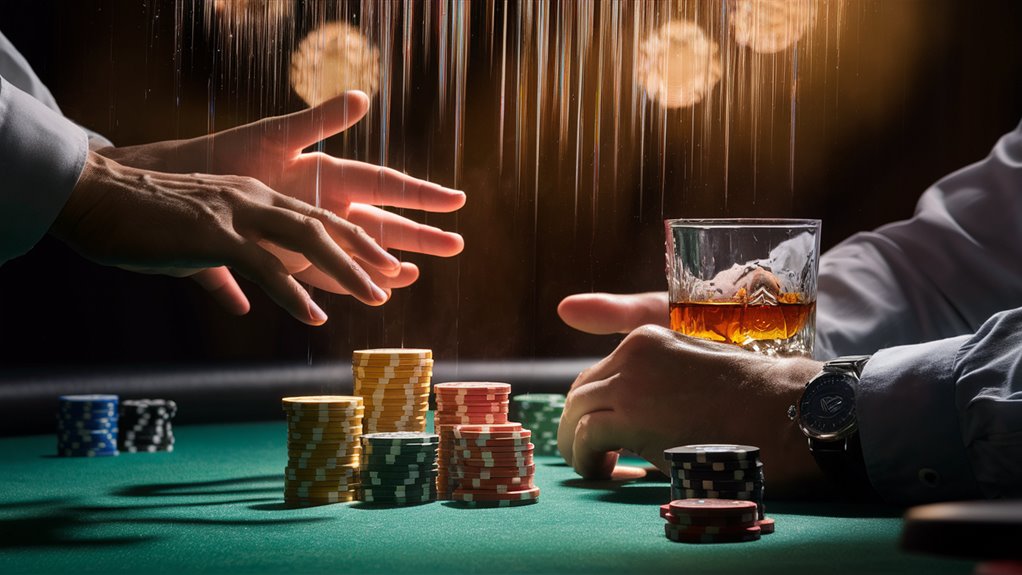
Analyzing Opponent Tendencies From Limited Data: A Statistical Approach
Understanding Player Patterns With Small Sample Sizes
Statistical analysis of poker opponents remains powerful even with limited data samples.
Through focused examination of just 30-50 hands, analysts can extract meaningful behavioral patterns and construct reliable player profiles by concentrating on key decision points.
Key Areas for Analysis
Preflop Tendencies
Late position raising frequencies serve as crucial baseline indicators of aggression levels. These patterns typically maintain consistency even across smaller samples, providing valuable insight into opponent strategy.
Post-Flop Behaviors
Tracking continuation betting frequencies and bet sizing choices across various board textures reveals exploitable tendencies.
Players generally demonstrate consistent decision-making in these spots, making them valuable data points even with limited exposure.
High-Leverage Situations
Focus on:
- 3-bet response patterns
- River betting frequencies
- Showdown tendencies
- Position-based statistics
- Stack depth correlations
Frequently Asked Questions
Q: How many hands are needed for reliable analysis?
A: 30-50 hands can provide statistically significant insights when focusing on key decision points.
Q: Which statistics matter most in small samples?
A: Late position raising, continuation betting frequencies, and responses to 3-bets offer the most reliable data.
Q: How can you minimize variance in small sample analysis?
A: Weight recent hands more heavily and focus on consistent situational patterns.
Q: What’re the most important positions to track?
A: Late position plays provide the clearest indication of baseline aggression levels.
Q: How should bet sizing tells be interpreted?
A: Analyze sizing patterns across different board textures and stack depths for consistent tendencies.
Statistical Analysis During Uncertainty
Statistical Analysis Under Uncertainty: A Comprehensive Guide
Understanding Probability in Complex Scenarios
Statistical analysis during periods of uncertainty requires sophisticated approaches to probability assessment and variance management.
Conditional probability tracking across multiple decision points enables navigation through unclear scenarios, particularly when working with incomplete information sets and undefined ranges.
Advanced Expected Value Calculations
The foundation of effective statistical decision-making lies in calculating expected value (EV) through dynamic probability distributions rather than static numbers.
When facing uncertain variables, implementing weighted probability assignments across different ranges provides more robust analytical frameworks. This approach enables:
- Multi-scenario simulation modeling
- Real-time probability adjustments
- Range-based analysis optimization
Bayesian Analysis Framework
Bayesian updating serves as a critical tool for handling limited data sets and evolving situations. This methodology involves:
- Starting with population-level baseline data
- Incorporating specific instance information
- Continuous probability revision based on new evidence
## Frequently Asked Questions
How do you calculate expected value under uncertainty?
Expected value calculations should incorporate multiple probability distributions and weighted scenarios rather than single-point estimates.
What role does Bayesian analysis play in statistical decision-making?
Bayesian analysis enables continuous probability updates as new information becomes available, improving decision accuracy over time.
How can you handle small sample sizes effectively?
Combine baseline population statistics with specific instance data while maintaining wide confidence intervals for more reliable analysis.
What’re the key components of range-based analysis?
Range-based analysis includes probability distribution mapping, scenario modeling, and dynamic adjustment based on observed patterns.
How do you maintain statistical accuracy with incomplete information?
Implement probabilistic frameworks that account for information gaps while continuously updating assumptions based on new data points.
Leveraging Incomplete Betting Information
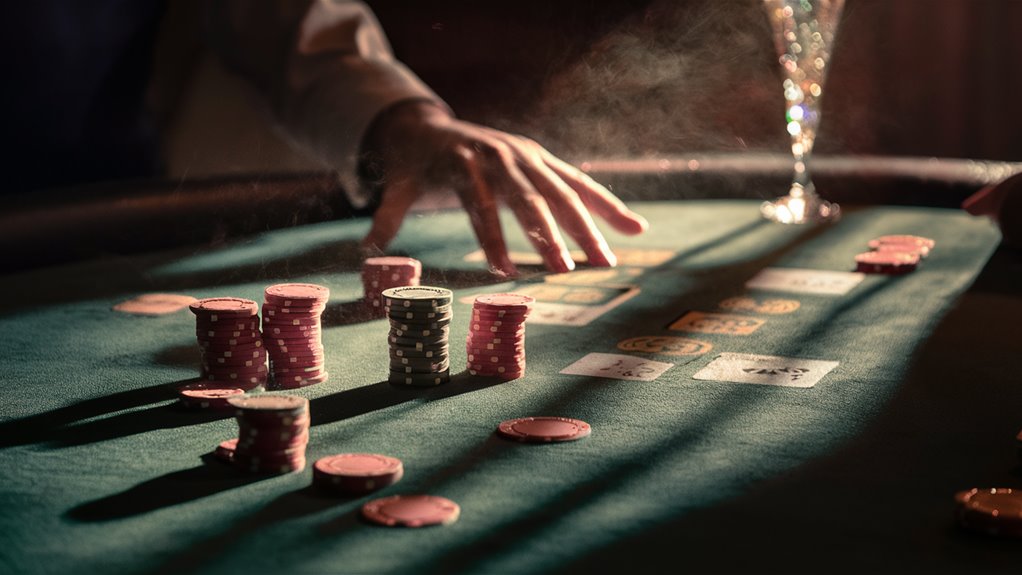
Maximizing Value from Incomplete Betting Data Analysis
Understanding Partial Information Strategy
Statistical analysis of betting patterns remains powerful even with incomplete data.
Through systematic probability mapping and advanced modeling techniques, analysts can extract meaningful insights from partial datasets to gain a competitive edge.
Leveraging Limited Betting Information
Key components for analyzing fragmented betting sequences:
- Baseline frequency establishment for known actions
- Probability range construction for missing data points
- Bayesian inference application for tendency estimation
- Board texture correlation with betting frequencies
Advanced Analysis Methods
Professional analysts utilize proxy indicators to strengthen incomplete data analysis:
- Bet sizing patterns relative to stack depths
- Timing correlations with hand strength ranges
- Positional tendencies across sample sets
- Integration with game theory optimal (GTO) baselines
FAQ: Incomplete Betting Analysis
Q: How much data is needed for reliable analysis?
A: While more data is better, meaningful patterns can emerge from as little as 40% of total hands when properly analyzed.
Q: What’re the most important indicators to track?
A: Bet sizing patterns, timing tells, and positional tendencies provide crucial insights even in incomplete datasets.
Q: How can missing data be accounted for?
A: Through Bayesian inference and probability mapping, analysts can construct reliable ranges for unknown variables.
Q: What role does GTO play in incomplete data analysis?
A: GTO principles provide baseline assumptions that help validate and strengthen partial data models.
Q: How accurate are predictions from incomplete data?
A: When properly analyzed using multiple indicators, predictions can achieve significant accuracy despite information gaps.